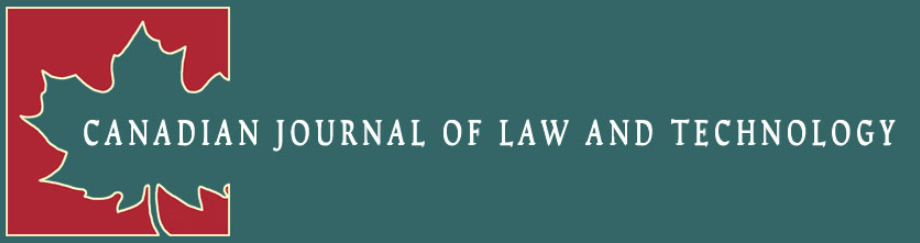
Keywords
terrorism, digital intermediaries, censorship, race and surveillance, islamic ideology, religious ideology, machine learning
Abstract
Post 9/11, where the current social and cultural temperature has constructed Islam as interchangeable with terrorism, digital intermediaries have responded with increased censorship of speech related to, emerging from, or advocating Islamic ideology. At the heart of this paper is the argument that digital intermediaries have relied on the opaqueness of machine learning technology (‘‘MLT”) to realize racialized surveillance, whereby speech concerning Islamic content has been disproportionally censored. This paper maps out how inherent biases concerning the ideology of Islam have been interwoven into the coding and machine learning used by the major tech giants. As a result of the algorithmic opacity of machine learning, not only has there been an increased targeting of speech affiliated with this religious ideology (and, by extension, the ethnic group that follows this religious ideology), but a disquieting opportunity has arisen for the broad censorship of content that lacks a meaningful or decisive link to violence. In addition to mapping out the intersection between race and surveillance in MLT, this paper also offers suggestions as to how to bolster algorithmic hygiene in MLT.
Recommended Citation
Subhah Wadhawan, "Let the Machines Do the Dirty Work: Social Media, Machine Learning Technology and the Iteration of Racialized Surveillance" (2022) 20:1 CJLT 1.
Included in
Computer Law Commons, Intellectual Property Law Commons, Internet Law Commons, Privacy Law Commons, Science and Technology Law Commons